Product Management & Operations
The success of a product doesn’t just depend on a good idea, but on its execution, led by a Product Manager (PM) and supported by other product profiles (Product Designer, Tech Lead, Researchers, Data Analysts…). Adopting a strategic, user-centered approach enables companies to enhance their ability to innovate and compete. The role of the PM is crucial for the creation and sustainable success of products and services, as it focuses on deeply understanding customers and solving real problems.
1. Product Roles and Functions
Roles in Product Management are neither singular nor static — they can take different shapes depending on the type of product, its stage of development, or the structure of the organization. In this section, we explore the different levels of seniority within a product team, from more tactical roles to those with a global strategic vision. Understanding these distinctions helps us properly scale teams and identify the type of leadership each project needs.
Roles within Product Management
The functions of a Product Manager, depending on the product’s scope, can be more strategic or more tactical. There are different seniority roles associated with product management. From lower to higher responsibility and strategic vision, these are some of the main roles:
- Product Manager (PM)
- Lead Product Manager / Tribe Lead (Lead PM)
- Head of Product (HoP)
- Chief Product Officer (CPO)
At mendesaltaren, we define the team with these roles based on the project’s needs. For example, we may need an CPO Advisor for companies that need to establish product processes, train teams, and get the team running. This role is also very key for new products that need to define their strategy and vision.
However, for other products, we might need a Product Manager, leading a work team in a specific vertical that requires additional support or our expertise in a particular area.
.png)
Key Roles of a Product Manager
A Product Manager (PM) will have different responsibilities depending on the position within the organizational structure as well as their experience, however their main responsibilities are:
- Defining the product vision and strategy (and its execution): The PM sets short- and long-term goals to guide the product’s evolution and align teams toward achieving them.
- Managing the product lifecycle: Thet encure that objectives are met at each stage of the product lifecycle, prioritizing features and iterations at the right times.
- User and market research: The PM analyzes product data and competitor insights to understand customer needs and behaviors, aligning them with business goals.
- Aligning and collaborating with multiple teams: Acts as a bridge between development, design, marketing, and sales, ensuring effective communication and alignment of objectives.
- Evaluating performance: Through key metrics like customer satisfaction, retention, and revenue growth, the PM evaluates product performance to make data-driven decisions.
A Product Manager has many responsibilities that drive a continuous cycle of product improvement. In the next section, we will continue with the concept of Continuous Discovery—a process that spans across everything. This process is complemented by constant validation, the development of a product mindset in teams, and precise analytics to measure and optimize performance. Let’s get started…
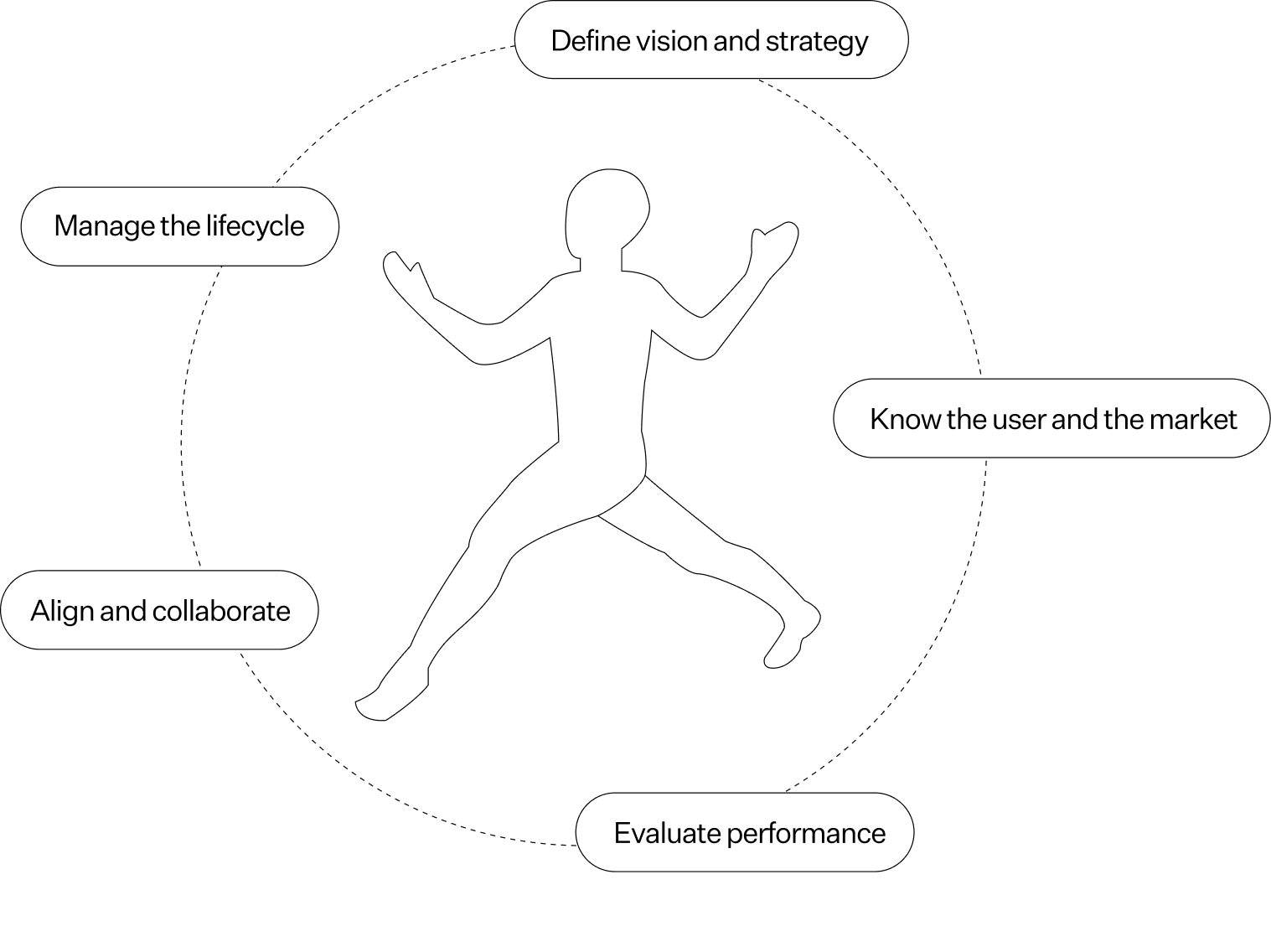
2. Continuous Discovery
Continuous Discovery instills the idea that product learning and research should never stop. The term was coined by Teresa Torres and is a philosophy we aim to instill in our clients’ organizations. There are always opportunities to improve, innovate, and find new solutions, by maintaining an open and curious mindset at all times.
The Difference Between a Discovery Process and Continuous Discovery
First, it’s important to highlight the key difference between a Discovery process and Continuous Discovery. Discovery, also known as the research phase, is a specific, time-bound initiative conducted at any stage of product maturity. Its goal is to understand user needs and define a value proposition.
However, the Continuous Discovery is an ongoing, cross-functional process that runs throughout the entire product lifecycle. It is more than just a phase—it is a mindset and approach, supported by structured processes and artifacts.
Unlike traditional Discovery, Continuous Discovery does not stop after the initial phase. Instead, it enables teams to keep exploring, validating, and refining the product based on new insights and market changes. This ensures a constant evolution aligned with both real user needs and business objectives. At Mendesaltaren, this philosophy is at the core of how we approach product management.

Implementation
We commonly get asked how can clients integrate this into their organization — we’ll explore our approach. In these projects, we design and implement workflows and dynamics that enhance and streamline the discovery of new insights, the generation of hypotheses, and their validation. This, in turn, allows them to validate their idea, product, business model, or simply scale it.
We start by analyzing the situation and, based on the client’s target expertise, product maturity, or available resources, we support and train different teams — from C-Level to more technical profiles — we dive into the areas that will make them more agile, critical, autonomous, and versatile in shaping their strategy and iterating their product.
We work closely with internal teams, providing tailored training, facilitating strategic workshops, and ensuring a smooth integration into the organizational culture. With this methodology, they strengthen their ability to innovate and adapt in a dynamic market.
- Process Design: We implement processes that facilitate the rapid and continuous generation and validation of hypotheses.
- Workshops & mentoring: We conduct strategic sessions and integrate into the organizational culture to ensure Discovery dynamics are sustained over time.
- Training: We support internal teams (from C-Level to technical profiles) to improve agility and autonomy in decision-making.
📎 Practical example: A product team holds biweekly brainstorming sessions to explore new features and validates them through A/B testing before implementation.
The Product Mindset: Embedding a Product Culture in Teams
A significant part of continuous discovery work is embedding a product culture within the client’s organization. A product mindset enables teams to ask the right questions and adopt a collaborative attitude. Instilling this culture is a daily effort that starts at a strategic level, and we apply it both within mendesaltaren and in our clients’ teams to empower them once the handover takes place.
Based on our experience, the best ways to achieve this include:
- Continuous learning: Internally, we conduct product demos, provide cross-functional knowledge sharing, and follow external training programs for our team. Externally, we offer tailored training sessions for product, design, and data teams.
- Encouraging collaboration and knowledge sharing: We create spaces where we share learnings and discoveries from both successes and failures so that everyone can benefit.
- Aligning the entire company with the vision: We ensure teams understand the “what,” “how,” and “why” of each initiative to provide visibility, foster synergies, and keep them motivated.
- Celebrating learning and achievements: We recognize discoveries and progress made by the team to maintain enthusiasm and engagement.
- Using cross-functional tools: We integrate artifacts like Product Mindset Questions into the team’s daily routines to encourage critical thinking when tackling any task.
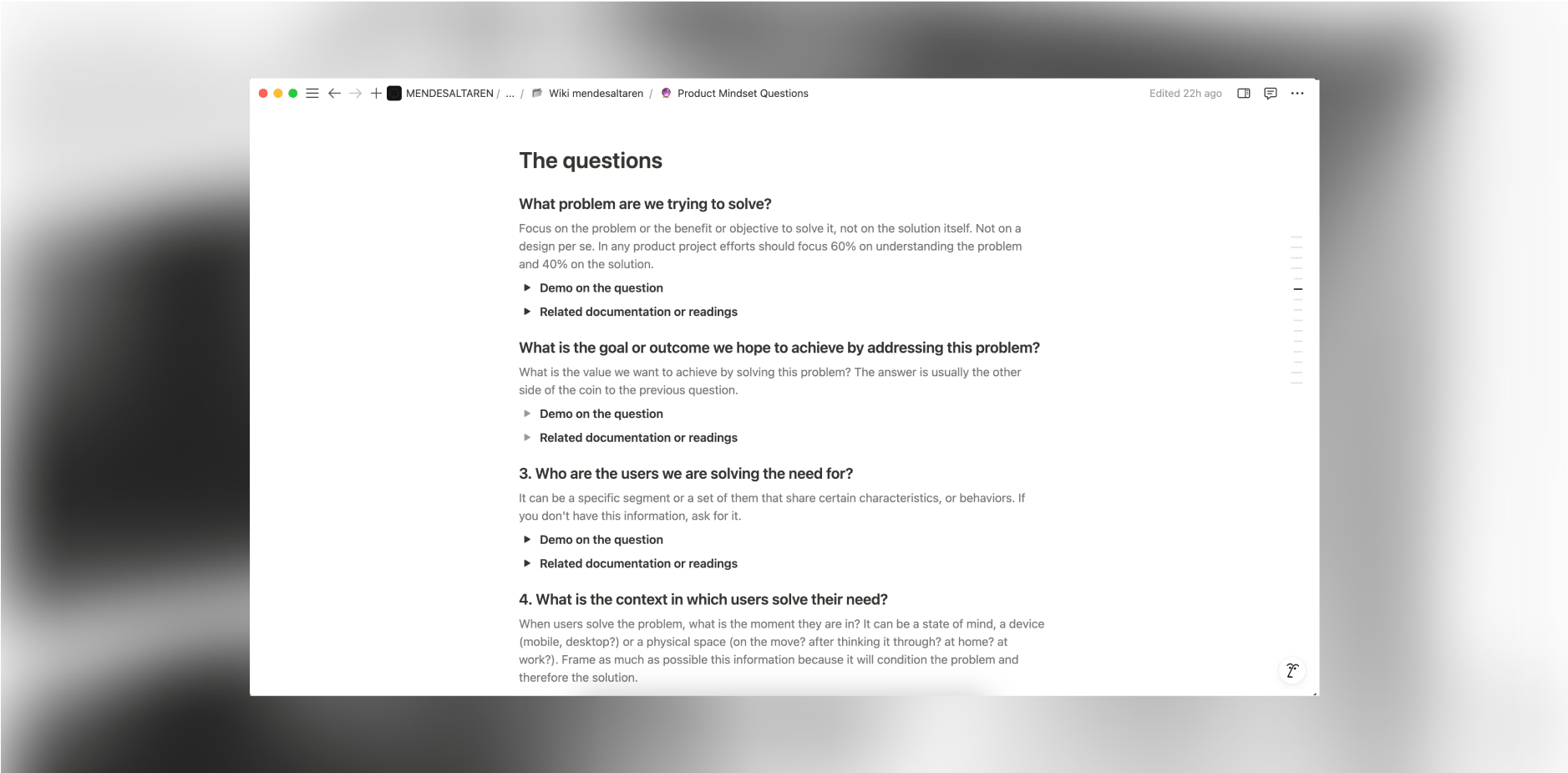
3. Validating Your Hypothesis
The goal of a launch is to validate or refute our business hypothesis or new functionality and continue improving the product. To achieve this, we use techniques applicable to both ideas and prototypes or MVPs. For this, we apply two main frameworks:
Problem-Solution Fit: Are We Solving a Real Problem?
We need to ensure that we have understood the users’ needs and problems, and that our proposal effectively addresses them. This helps us avoid developing products without real demand.There are various tools to validate this, such as the Problem-Solution Fit Canvas from Lean Startup, developed by Daria Nepriakhina, prototypes, focus groups, and user testing. The specific approach will be decided based on the context.
Product-Market Fit: Does the Market Need This Product?
Building a product means, to some extent, accepting uncertainty. Everything has an experimental nature, and our experience, intuition, and research work will all play equally fundamental roles. There is no foolproof formula or data that tells us the most effective path to validate our hypotheses or when we should stop investing in our idea. The red lines are usually defined by what we call the “business appetite,” meaning what the company is willing to invest and its constraints.
To find our product’s fit, we first need to ask ourselves: How do we define the success of our product? How do we know when we’ve achieved market fit? What timeline do we have, and which metrics will we use to measure it?
To answer these questions, we need what we call a validation framework. A validation framework is the definition of a timeline and set metrics to regularly monitor the evolution of our product until we can confidently say it has achieved product-market fit.
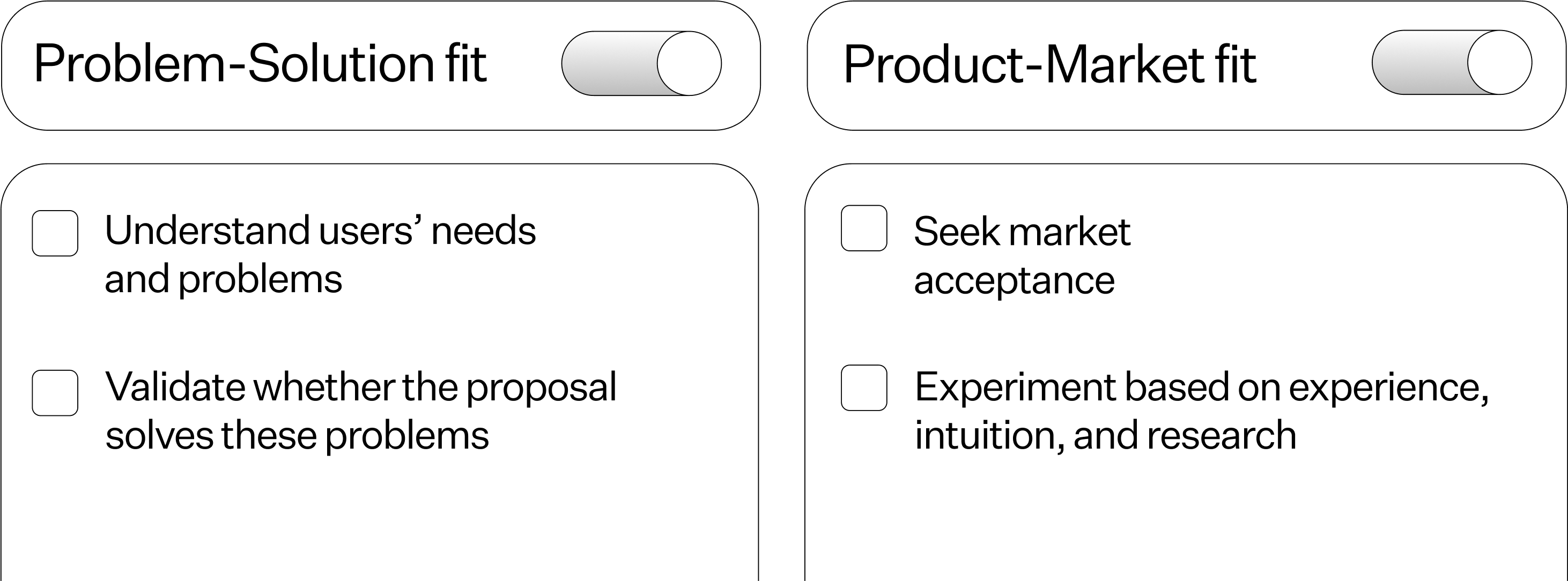
Metrics to Validate Product-Market Fit
As we mentioned earlier, metrics will be one of the key variables that help us validate Product-Market Fit. While metrics vary greatly depending on the product, there are some common business indicators that are frequently used:
- MRR (Monthly Recurring Revenue)
- CAC (Customer Acquisition Cost)
- DAU/WAU/MAU (Daily/Weekly/Monthly Active Users)
- Retention and/or Stickiness
- Conversions
Monitoring the digital product’s performance in the market based on these metrics, along with other insights from qualitative research, will help us improve the product, adjust the marketing strategy, and optimize the tactics used in the go-to-market approach.
Timeline to Validate Product-Market Fit
Many articles and books discuss the maximum timeframes within which we should be able to validate or discard our product hypothesis, but the truth is that each sector and idea has its own unique constraints.
The timeline we set to validate our product will depend heavily on multiple factors: business appetite, technical and marketing constraints, the achievement of key milestones to implement the Go-To-Market strategy, and more.
Is a 6-month timeframe acceptable when we don’t have the means to implement the Go-To-Market strategy and reach the necessary users to understand if we are solving their problem? Is a 1-year timeframe acceptable when we haven’t been able to develop the key functionality because we don’t have the right team?
There are many variables where the perfect formula doesn’t exist, so the timeline can be flexible. We can set a maximum validation period with periodic reviews to make decisions or adjust the timeline based on our progress and constraints.
To achieve market fit for our product or service, we need to validate each improvement we make quickly, and this is where continuous discovery comes into play.

4. Product Analytics Strategy
To understand a product’s performance, user engagement, and its impact on business goals, a product analytics strategy allows us to design, implement, collect, and interpret related data. How should we approach quantitative data? Where should we start familiarizing ourselves with it? What are the most important KPIs? What should I focus on when approaching a redesign?
Having a good product analytics strategy aligned with business objectives will help reduce uncertainty about your product, validate ideas, and iterate your product faster
Key Objectives of Product Analytics
Product analytics has three main objectives:
- Explore: Extract insights to propose improvements and discover opportunities.
- Monitor: Detect anomalies or unexpected behaviors.
- Validate: Measure the success of specific features or iterations.
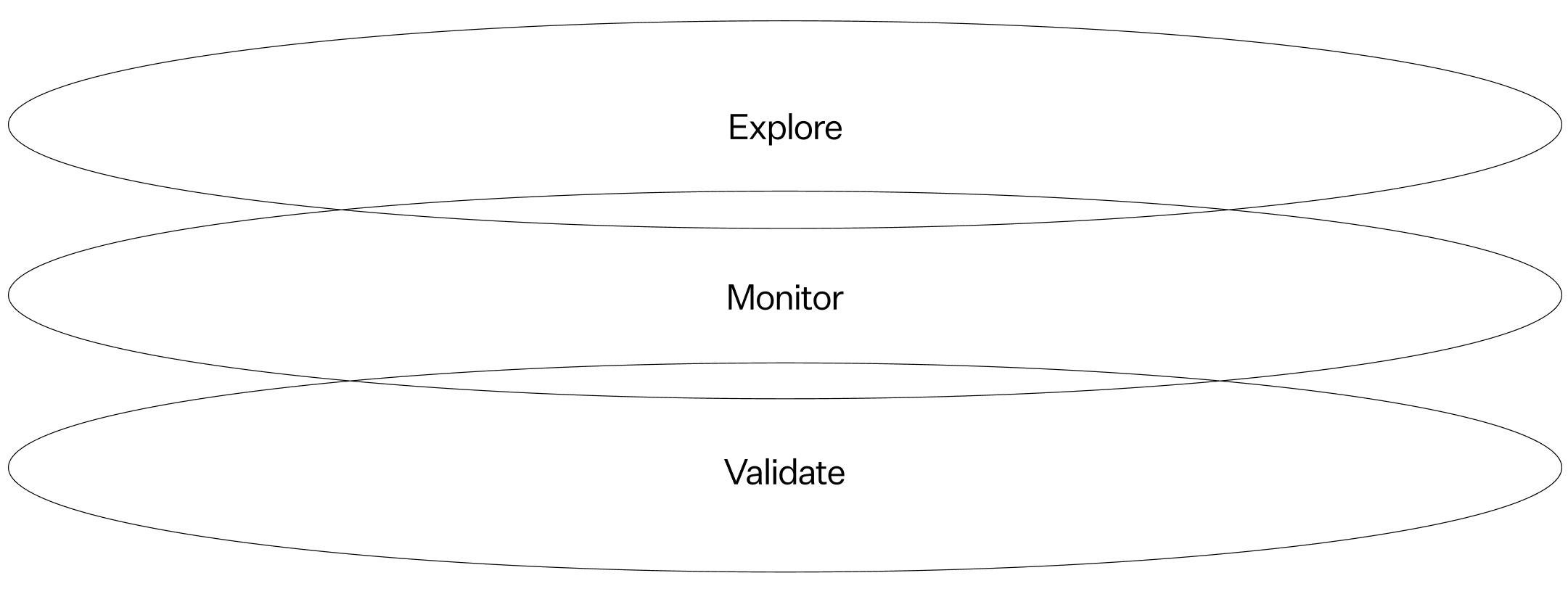
Approaches to Data Analysis
Once we have gathered our data, both from product analytics and other quantitative sources such as surveys, questionnaires, or server logs, we can approach it from different perspectives, depending on the weight we give it in our decisions:
- Data-Driven Design: Where data dictates the strategy. This approach is based entirely on the data extracted from product analysis, without considering any other insights.
📎 Practical Example: A PM notices that the checkout conversion rate has dropped by 15%. Without considering qualitative feedback, they implement the design with the best performance based on previous A/B tests, as the data indicates it’s the most effective option.
- Data-Informed: Where data is considered, but not the sole driver. This approach takes into account not only the data but also past experience, professional intuition, reasoning, judgment, and feedback from other stakeholders. It’s a perfect blend of context and both quantitative and qualitative data.
📎 Practical Example: A PM analyzes retention metrics and user feedback. Although the data suggests that a new feature isn’t being heavily used, the team decides to iterate on its design rather than remove it because they believe that with adjustments to the UX, it can gain more adoption. Additionally, the limited feedback it receives is exceptionally good.
- Data-Inspired: Where data inspires actions. Trends and patterns are sought across multiple data sources, but decisions are not solely based on these data. The data is used to generate insights and inspire new ideas and strategies without expecting a scientific or mathematical result, but rather an experimental one.
📎 Practical Example: A PM discovers a trend study that shows, with statistics, that in various industries, users interact more with conversational interfaces. The team decides to open the discussion on which types of conversational solutions and at which points in the flow would be interesting to develop to improve user experience in the app.
All three approaches foster curiosity about data across the organization, while the subjective element of human interpretation remains in all three. Therefore, the key takeaway is that none of these approaches possess absolute truth. The best thing we can do to build a solid product strategy is to choose the approach—or combination of approaches—that is most appropriate for each initiative.

Implementation Scenarios
There are three scenarios where having a solid data strategy and applying product analytics is especially useful:
- When creating a product from scratch: We apply a comprehensive strategy by developing a measurement plan aligned with business goals and a tagging guide to instrument the product.
📎 Practical Example: We’re creating a padel court booking app. A PM with data skills should understand the macro goals (space reservations) and micro-goals (user registrations, space queries, booking management, etc.), devise an appropriate measurement plan, and ensure the tagging guide covers all of these aspects. - When redesigning a product: We analyze a product from multiple angles, including analytics, to understand what works and what doesn’t, and bring those insights into the new product we’re working on.
📎 Practical Example: We’re redesigning the product page of a clothing ecommerce site to increase the add-to-cart rate. To do this, we’ll review data we have regarding it: Do users often use the size guide? Does the cart content change significantly after adding products? Why? Do users add products from the related products modules? - When iterating a product: Here, we need to propose hypotheses and solutions and validate or refute them. We’ll use product analytics and research on the issue to complete the hypothesis. 📎 Practical Example: A PM detects that the conversion rate of a form has dropped. Upon analyzing the data, they discover that a change in the interface is negatively affecting the user experience, leading to an immediate correction.
Additionally, product analytics serves as support and guidance to ensure that the product is being used normally or, in the case of anomalies, to detect issues. This is why it’s so important that all profiles involved in the product are data-sensitive.

Key Questions for Analytics
To wrap up, it’s essential to ask a series of fundamental questions that help maintain focus and alignment:
- What am I trying to improve?
- What do these data justify?
- Have we validated these data with qualitative information?
Conclusion
Effective product management not only ensures that the product meets user needs but also drives long-term growth and innovation. The combination of a clear strategy, continuous validation, a product mindset, and rigorous analytics enables companies to adapt to a dynamic and competitive market.